Segmentation of metallographic images based on improved CV model
-
摘要: 對金相圖像進行快速精確分割是金相晶粒評級的關鍵步驟,利用傳統Chan-Vese(CV)模型很難將晶粒精確地提取出來.為了更加精確地對金相圖像進行分割,提出一種基于改進CV模型的金相圖像分割方法.初始化水平集函數,對曲線內外兩部分分別計算其倒數坎貝拉距離,并將該距離的大小作為擬合中心的權重系數,有效抑制了噪聲點對區域擬合中心準確性的影響;引入指數熵自適應調節曲線內外能量權重,減少固定能量權重對曲線演化的影響;同時加入距離規范項以避免水平集函數的重新初始化,加速該模型的收斂.實驗結果表明,與傳統CV模型、測地線活動輪廓模型、距離規范項的水平集模型以及偏置場修正水平集模型相比,所提方法分割出的金相圖像更加精確,分割效率較高且模型收斂性較好.Abstract: The segmentation of metallographic images plays a key role in grain grading, but it is difficult to extract grains accurately using the traditional Chan-Vese (CV) model. To segment metallographic images more accurately, a metallographic image segmentation method based on an improved CV model was proposed. First, the level set function was initialized, and its reciprocal Canberra distance from inside and outside the curve was calculated. Then, these distances were used as weight coefficients of the fitting centers to restrain the influence of noise points on their accuracy. In addition, adding exponential entropy to adjust the energy inside and outside the curve reduces the influence of the fixed energy weight on the evolution of the curve. Lastly, to accelerate the convergence of the model, a distance-regularized term was introduced to avoid re-initialization of the level set function. The experimental results show that, compared with the traditional CV model, the geodesic active contour model, the distance-regularized level set evolution model, and the bias level correction level set model, the segmentation of the metallographic images based on the proposed model is more accurate and efficient, and the proposed model has better convergence.
-
參考文獻
[5] Chan T F, Vese L A. Active contours without edges. IEEE T Image Process, 2001, 10(2):266 [6] Li C, Kao C Y, Gore J C, et al. Minimization of region-scalable fitting energy for image segmentation. IEEE T Image Process, 2008, 17(10):1940 [9] Chen Y F, Liang H Q, Yue X D, et al. Active contour model with entropy-based constraint for image segmentation//SAI Computing Conference. London, 2016:259 [11] Caselles V, Kimmel R, Sapiro G. Geodesic active contours. Int J Comput Vision, 1997, 22(1):61 [12] Li C M, Xu C Y, Gui C F, et al. Distance regularized level set evolution and its application to image segmentation. IEEE T Image Process, 2010, 19(12):3243 [13] Li C, Gatenby C, Wang L, et al. A robust parametric method for bias field estimation and segmentation of MR images//IEEE Conference on Computer Vision and Pattern Recognition. Anchorage, 2009:218 [14] Li C M, Huang R, Ding Z H, et al. A level set method for image segmentation in the presence of intensity inhomogeneities with application to MRI. IEEE T Image Process, 2011, 20(7):2007 [15] Kass M, Witkin A, Terzopoulos D. Snakes:active contour models. Int J Comput Vision, 1988, 1(4):321 [20] Song Y, Wu Y Q, Dai Y M. A new active contour remote sensing river image segmentation algorithm inspired from the cross entropy. Digit Signal Process, 2016, 48:322 [21] Wang H, Huang T Z, Xu Z, et al. A two-stage image segmentation via global and local region active contours. Neurocomputing, 2016, 205:130 [22] Deng Y H, Liu X L, Zheng Z H, et al. A new active contour modeling method for processing-path extraction of flexible material. Optik-Int J Light Electron Opt, 2016, 127(13):5422 -
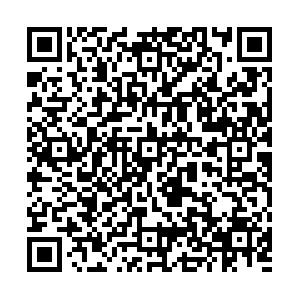
計量
- 文章訪問數: 832
- HTML全文瀏覽量: 287
- PDF下載量: 24
- 被引次數: 0