An outlier detection algorithm based on a soft hyper-sphere for high dimension nonlinear data
-
摘要: 在冶金、化工等流程型工業領域,生產中的過程控制參數往往具有高維非線性結構特征.為了解決這類高維復雜數據的異常點檢測問題,本文引入了軟超球體的概念,采用非線性核函數將原始數據映射到高維的特征空間,并在特征空間中確定軟超球體的邊界.通過檢測待識別樣本映射到特征空間的位置信息來判定過程參數的設定值是否為異常點,從而避免出現批量的產品質量問題.以某類汽車用鋼為應用實例,對實際生產數據進行檢測,證明了所提出的基于軟超球體的異常點識別算法對于高維的非線性數據具有良好的檢測能力.Abstract: In process industries, such as metallurgy and chemistry, real procedure parameters usually possess high-dimensional nonlinear features. To solve the problem of outlier detection in complex high-dimensional data, the concept of a soft hyper-sphere is introduced in this paper. An original data set is projected into a high-dimensional feature space using a nonlinear kernel function, and the boundary of the soft hyper-sphere is determined within this feature space. To avoid a mass product quality incident, location information on the testing samples, which are projected into the feature space, is used to decide whether they are outliers. As an applied example, practical procedure data obtained from a type of auto steel product were tested. The results verify that the proposed outlier detection algorithm based on a soft hyper-sphere has a better ability for outlier detection in high-dimensional nonlinear data than tradional methods.
-
參考文獻
[1] Zimek A, Schubert E, Kriegel H P. A survey on unsupervised outlier detection in high-dimensional numerical data. Statist Anal Data Min ASA Data Sci, 2012, 5(5):363 [2] Guo J H, Huang W, Williams B M. Real time traffic flow outlier detection using short-term traffic conditional variance prediction. Transport Res C Emerg Technol, 2015, 50:160 [6] Johnson J E. A User's Guide to Principal Components. New York:John Wiley&Sons Inc, 1991 [7] Wold S, Martens H, Wold H. The multivariate calibration problem in chemistry solved by the PLS method. Matrix Pencils, 1983, 973:286 [8] Tax D M J. One-Class Classification[Dissertation]. Dutch:Delft University of Technology, 2001 [9] Liu B, Xiao Y, Cao L, et al. SVDD-based outlier detection on uncertain data. Knowl Inform Syst, 2013, 34(3):597 [10] Sakla W, Chan A, Ji J, et al. An SVDD-based algorithm for target detection in hyperspectral imagery. IEEE Geosci Remote Sens Lett, 2011, 8(2):384 [11] Shawe-Taylor J, Cristianini N. Kernel Methods for Pattern Analysis. England:Cambridge University Press, 2004 [12] Rosipal R, Trejo L J. Kernel partial least squares regression in reproducing Kernel Hillbert space. J Mach Learn Res, 2001, 2:97 [13] Bach F R, Jordan M I. Kernel independent component analysis. J Mach Learn Res, 2002, 3:1 -
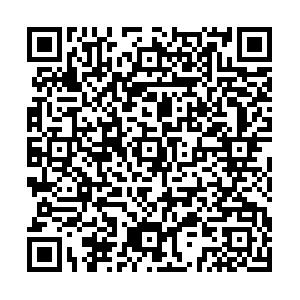
計量
- 文章訪問數: 717
- HTML全文瀏覽量: 243
- PDF下載量: 12
- 被引次數: 0