Bio-inspired motion-adaptive estimation algorithm of sequence image
-
摘要: 針對傳統算法在抗光照變化影響、大位移光流和異質點濾除等方面的不足,從人類視覺認知機理出發,提出了一種基于機器學習和生物模型的運動自適應V1-MT (motion-adaptive V1-MT,MAV1MT)序列圖像光流估計算法.首先,引入基于ROF模型的結構紋理分解(structure-texture decomposition,STD)技術,有效解決了光照和色彩變化的影響.其次,利用多V1細胞加權組合及非線性正則化模擬MT細胞模型,并結合嶺回歸訓練學習得到運動自適應的權重,解決對目標的運動速度感知問題.最后,引入由粗到精的增強方法和圖像金字塔局部運動估計采樣,將V1-MT運動估計模型應用于實際大位移視頻序列.理論分析和實驗結果表明,新方法能更加擬合人眼視覺信息處理特性,對視頻序列具有普適、有效、魯棒的運動感知性能.Abstract: To overcome the insufficiencies of varying illumination, large displacement estimation, and outlier removal, a motion-adaptive V1-MT (MAV1MT) motion estimation algorithm based on machine learning and a bio-inspired model of sequence image was proposed, starting from the theory of visual cognition. First, a structure-texture decomposition technique based on the Rudin Osher Fatemi (ROF) model was introduced to manage the variation in illumination and color. Then, a pooling stage at the MT level with non-normalization, which combines the afferent V1 responses using the adaptive weights trained by ridge regression, is modeled to obtain the local velocities. Finally, through introducing the coarse-to-fine method and pyramid structure subsampling of the local motion, the MAV1MT model is used on realistic video. Theoretical analysis and experimental results suggest the new algorithm, which is more fitting to information processing features of the human visual system, has universal, effective and robust motion perception performance.
-
Key words:
- optical flow /
- V1 /
- MT /
- spatial-temporal filter /
- motion perception /
- ridge regression
-
參考文獻
[1] Fortun D, Bouthemy P, Kervrann C. Optical flow modeling and computation:a survey. Comput Vision Image Understanding, 2015, 134:1 [2] Horn B K P, Schunck B G. Determining optical flow. Artif Intelligence, 1981, 17(1-3):185 [3] Butler D J, Wulff J, Stanley G B, et al. A naturalistic open source movie for optical flow evaluation//12th European Conference on Computer Vision. Firenze, 2012:611 [4] Revaud J, Weinzaepfel P, Harchaoui Z, et al. Epicflow:edge-preserving interpolation of correspondences for optical flow//Proceedings of the IEEE Conference on Computer Vision and Pattern Recognition. Boston, 2015:1164 [6] Heeger D J. Optical flow using spatiotemporal filters. Int J Comput Vision, 1988, 1(4):279 [7] Rust N C, Mante V, Simoncelli E P, et al. How MT cells analyze the motion of visual patterns. Nat Neurosci, 2006, 9(11):1421 [8] Nishimoto S, Gallant J L. A three-dimensional spatiotemporal receptive field model explains responses of area MT neurons to naturalistic movies. J Neurosci, 2011, 31(41):14551 [9] Solari F, Chessa M, Medathati N V K, et al. What can we expect from a V1-MT feedforward architecture for optical flow estimation?. Signal Processing:Image Commun, 2015, 39:342 [10] Wedel A, Pock T, Zach C, et al. An improved algorithm for TV-L1 optical flow//Statistical and Geometrical Approaches to Visual Motion Analysis. Berlin, 2009:23 [12] Baker S, Scharstein D, Lewis J P, et al. A database and evaluation methodology for optical flow. Int J Comput Vision, 2011, 92(1):1 -
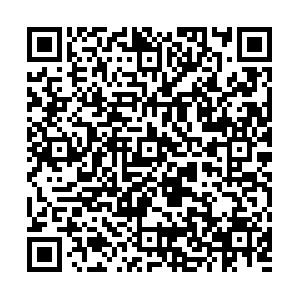
計量
- 文章訪問數: 766
- HTML全文瀏覽量: 271
- PDF下載量: 11
- 被引次數: 0