Similarity dynamical clustering algorithm based on multidimensional shape features for time series
-
摘要: 由于時間序列數據具有高維度、動態性等特點,這就導致傳統的數據挖掘技術很難有效的對其進行處理,為此,提出了一種基于多維時間序列形態特征的相似性動態聚類算法(similarity dynamical clustering algorithm based on multidimensionalshape features for time series,SDCTS).首先,提取多維時間序列的特征點以實現降維,然后,根據多維時間序列的斜率、長度和幅值變化的形態特征定義了一種新的時間序列相似性度量標準,進而提出無需人為給定聚類個數的多維時間序列動態聚類算法.實驗結果表明,與其他算法相比,此算法對時間序列具有良好的聚類效果.Abstract: Traditional data mining methods are difficult to deal with the high dimensionality and dynamics characteristic of the time series. Therefore, in this study, a similarity dynamical clustering algorithm based on multidimensional shape features for time series (SDCTS) was proposed. First, the feature points of multidimensional time series are extracted to realize dimensionality reduction. Second, a new similarity measure criterion is defined with the shape features (slope, length, and amplitude) of the obtained multidimensional time series, and thus a dynamical clustering algorithm of multidimensional time series is proposed without predefining clustering numbers. The experimental results demonstrate that the SDCTS algorithm improves the clustering accuracy for time series compared with other algorithms.
-
Key words:
- similarity measure /
- clustering /
- time series /
- shape features /
- dimensionality reduction
-
參考文獻
[3] Agrawal R, Faloutsos C, Swami A. Efficient similarity search in sequence databases//International Conference on Foundations of Data Organization and Algorithms. Chicago, 1993:69 [5] Keogh E J, Pazzani M J. A simple dimensionality reduction technique for fast similarity search in large time series databases//Pacific-Asia Conference on Knowledge Discovery and Data Mining. Kyoto, 2000:122 [8] Łuczak M. Hierarchical clustering of time series data with parametric derivative dynamic time warping. Expert Syst Appl, 2016, 62:116 [15] Izakian H, Pedrycz W, Jamal I. Fuzzy clustering of time series data using dynamic time warping distance. Eng Appl Artif Intelligence, 2015, 39:235 [17] Hung N Q V, Anh D T. An improvement of PAA for dimensionality reduction in large time series databases//Pacific Rim International Conference on Artificial Intelligence. Hanoi, 2008:698 -
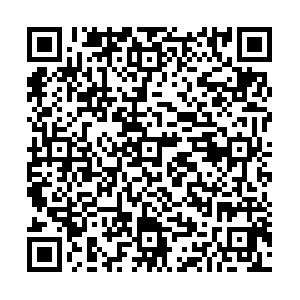
計量
- 文章訪問數: 1025
- HTML全文瀏覽量: 406
- PDF下載量: 60
- 被引次數: 0